Can you trust ChatGPT with your health? Why LLMs with doctor oversight are a safer bet
While natural language processing (NLP) undoubtedly comes with a wide range of advantages for the healthcare industry, the implications of using general large language model (LLM) applications like ChatGPT in healthcare can be risky. In this XUND Insight, we explore the dangers of utilizing black box models for health-related questions, and discuss why it is crucial to work with more focused NLP models and ones that follow strict quality control guidelines and are constantly double-checked by actual healthcare professionals.
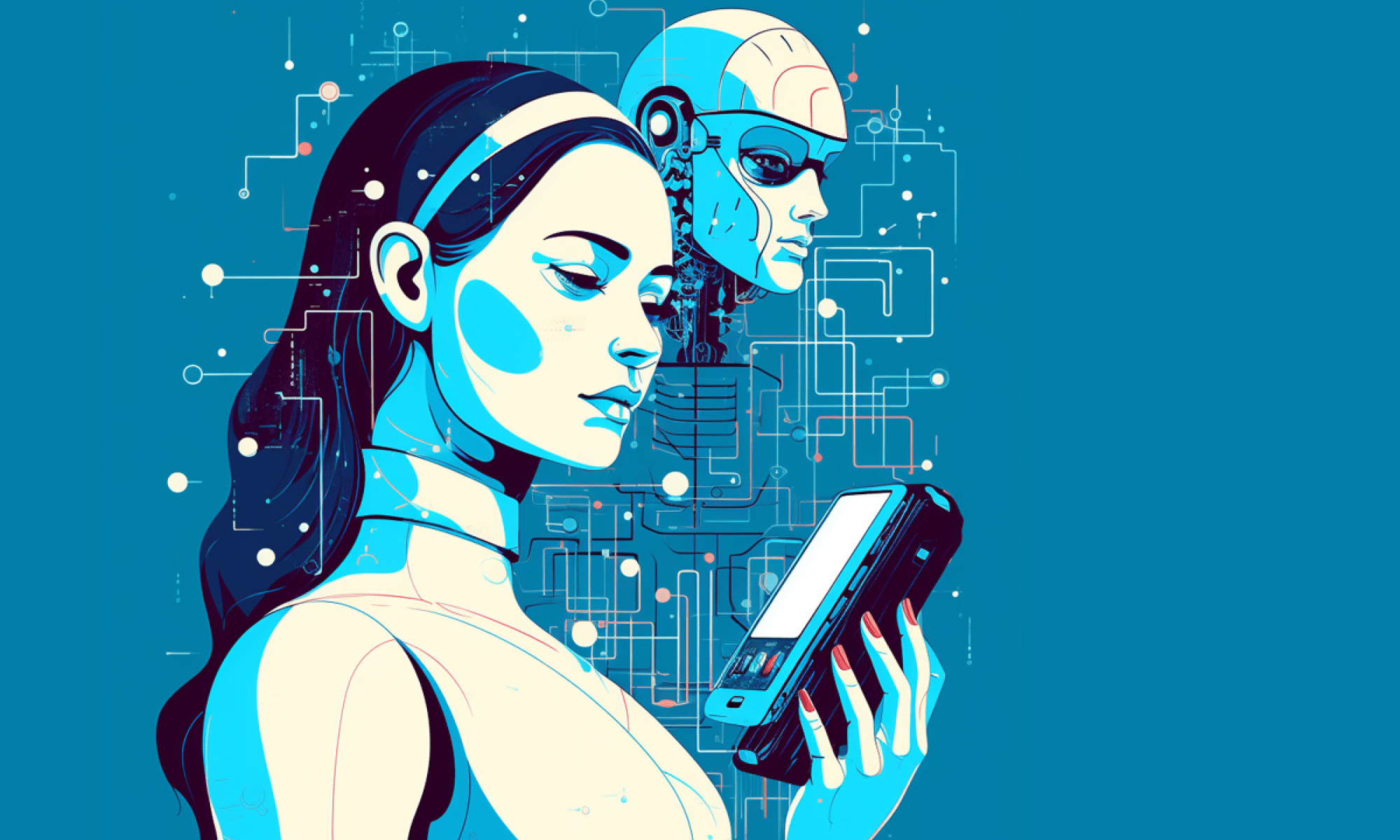
The rise of large language models (LLMs)
Truth be told, NLP has been around for quite some time, but it was only recently that people other than Data Scientists, and tech aficionados, became aware of NLP applications such as ChatGPT.
In fact, the early beginnings of NLP date back to the late 1940s, when there was a growing interest in computer-based machine translation. However, that early form of NLP vastly differs from what we have today. While there was more accelerated progress over the past few decades, the adoption amongst the user base had been staggered until recently.
Now, with the increased processing power of computers, and the gigantic volumes of data available, we are experiencing firsthand a new era in technological development: The mass adoption of LLMs by the general public. From specialists to lay people, there has been an ever-growing interest in the field of generative AI.
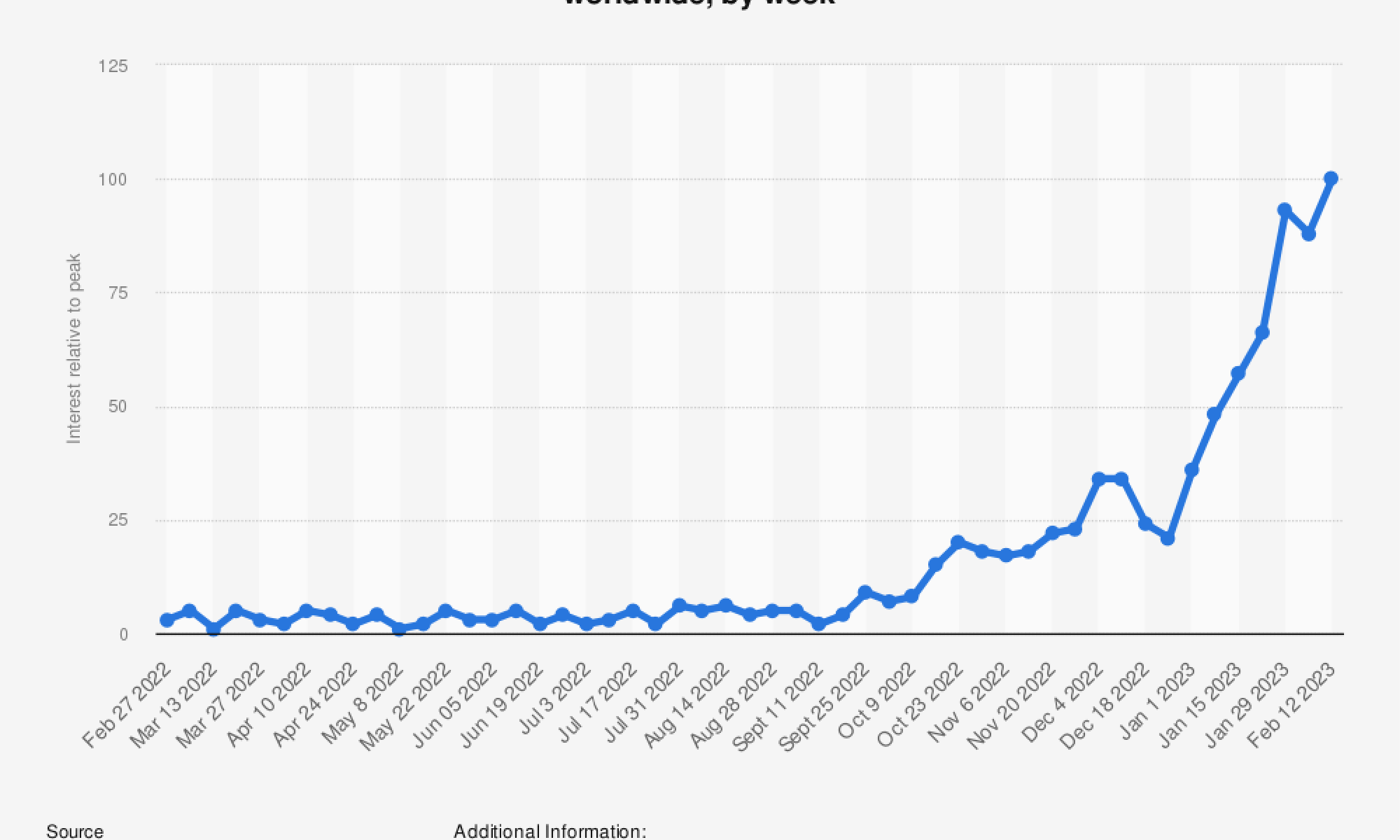
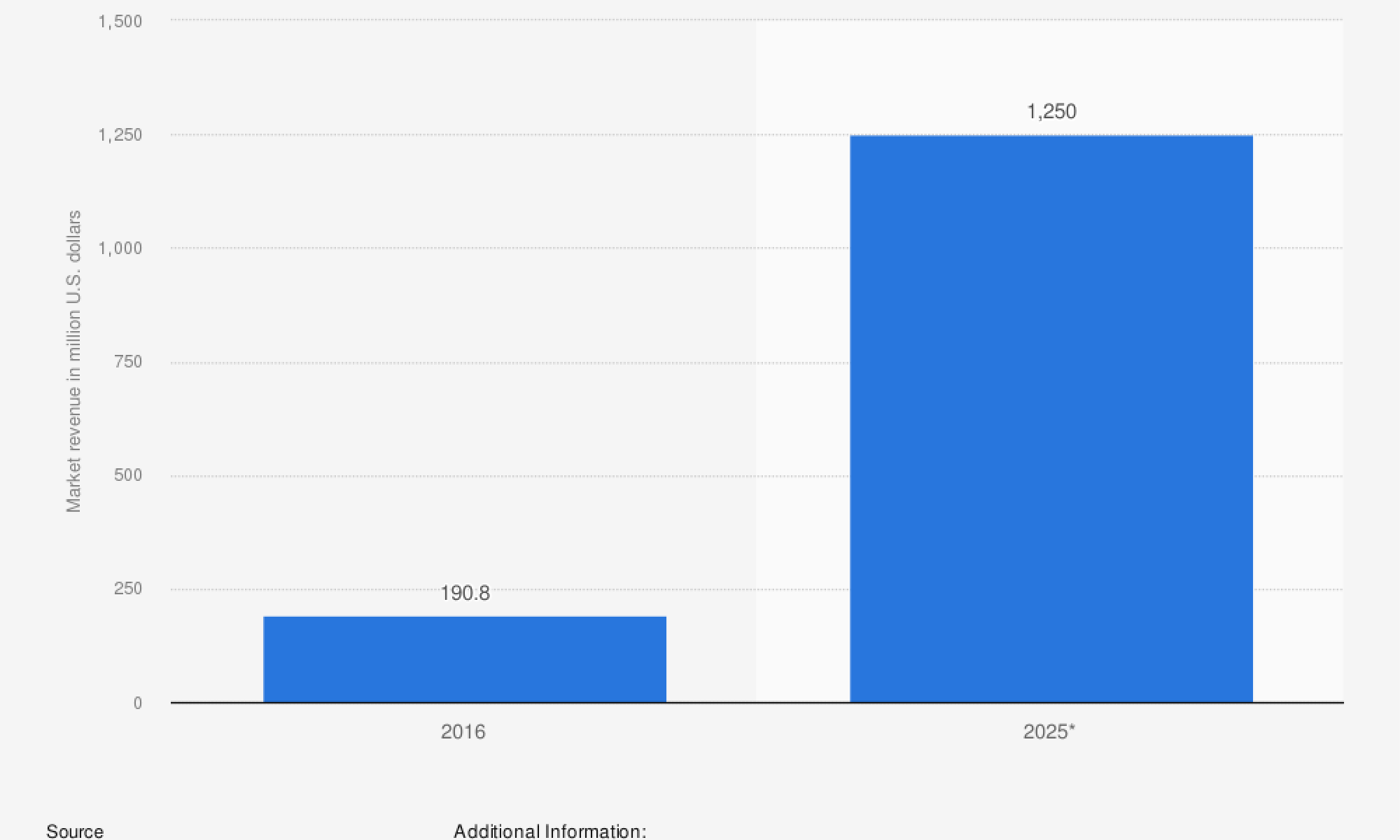
A brief introduction to ChatGPT
ChatGPT runs on a sophisticated pattern recognition technology and belongs to the latest generation of LLMs. It is a class of AI that is programmed to be able to perform deep learning, i.e., to improve itself with each user interaction and as online content continues to grow.
Informally called a chatbot, this generative pre-trained transformer is capable of mimicking a human conversation to such a degree that users are having a hard time identifying content that was written by AI as opposed to a human. The surge of users within the first few months since its public release at the end of November 2022 has been overwhelming: it had 1 million users within five days of its launch, and two months later, the active user base was estimated to be upwards of a 100 million users.
While a lot of people are using it for fun and out of curiosity, some users are also discovering the possibilities it holds for professional settings. As job demands and time constraints grow throughout a variety of different industries, saving time and working more efficiently becomes ever more important. It can even help with coding, content creation, or planning and scheduling, just to name a few. In fact, to some, ChatGPT can even serve as a chat partner to whom they can open up and ask for personal advice.
Use cases of ChatGPT in healthcare & medical research
Generative AI has the potential to revolutionize several industries, including healthcare. Use cases of ChatGPT in healthcare include:
- Medical recordkeeping & writing: Automatically generate summaries of patient interactions and medical histories, real-time assistance for writing and documenting medical reports, including clinical notes and discharge summaries.
- Medical translation: Real-time translation services to promote effective communication between healthcare providers and patients.
- Medical education: Students and healthcare professionals can have immediate access to pertinent medical information and resources, which can aid in their continual growth and development.
Overall, ChatGPT can potentially support healthcare professionals with repetitive and routine administrative tasks. However, some of its users might even ask the chatbot for medical advice. A recent investigation of researchers from the Johns Hopkins University and the University of California San Diego revealed that people preferred chatbot AI responses over replies given by physicians when it comes to both quality and empathy.
And herein lies one of the many pitfalls of LLMs such as ChatGPT in the context of healthcare: Sometimes, it can appear so convincingly human and knowledgeable that people trust its advice as much as they would a medical professional. Given the current state of the technology, this could lead to serious risks and harm.
How trustworthy is information from a black box?
The latest version of ChatGPT was trained on billions of pages of material from all across the internet, including social media platforms. The sheer size of GPT-4 and OpenAI’s lack of openness regarding the data sources it was trained on effectively make it an impenetrable black box of information. Although much of the machine learning itself is supervised, the countless responses it gives cannot all be scrutinized beyond user feedback, users who are knowledge seekers, not knowledge holders to begin with. And that user feedback is yet another new input into the black box.
It is not only difficult to discern where ChatGPT’s data is being sourced from but also the validity and trustworthiness of that data. So although it is capable of generating content in a variety of different fields, all of this comes with an unverifiable level of factuality, along with no way to filter out truth bias from its sources. There is even the risk of ChatGPT leaking parts of chat histories, or coming up with “hallucinations”, i.e., making false statements and even citing false or non-existent articles.
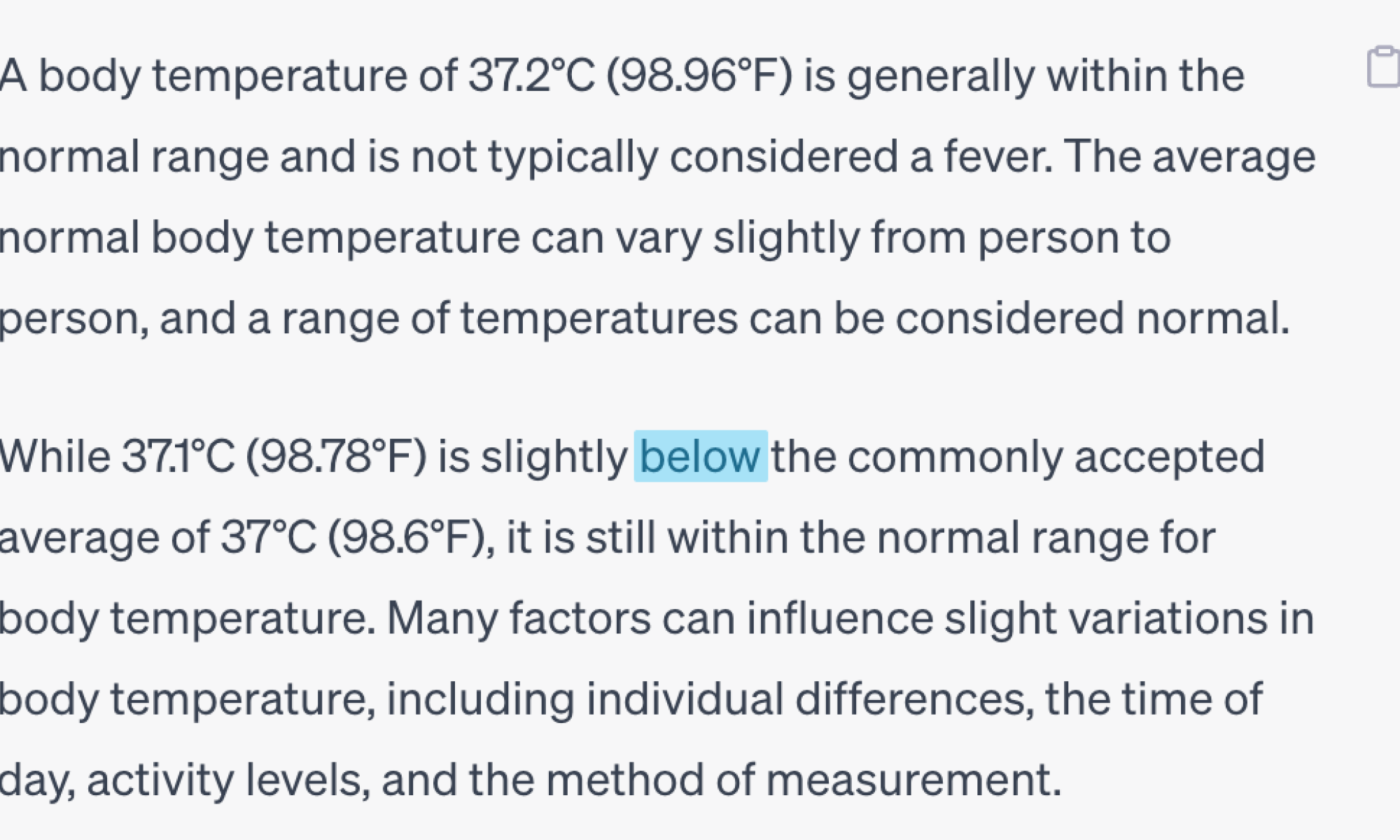
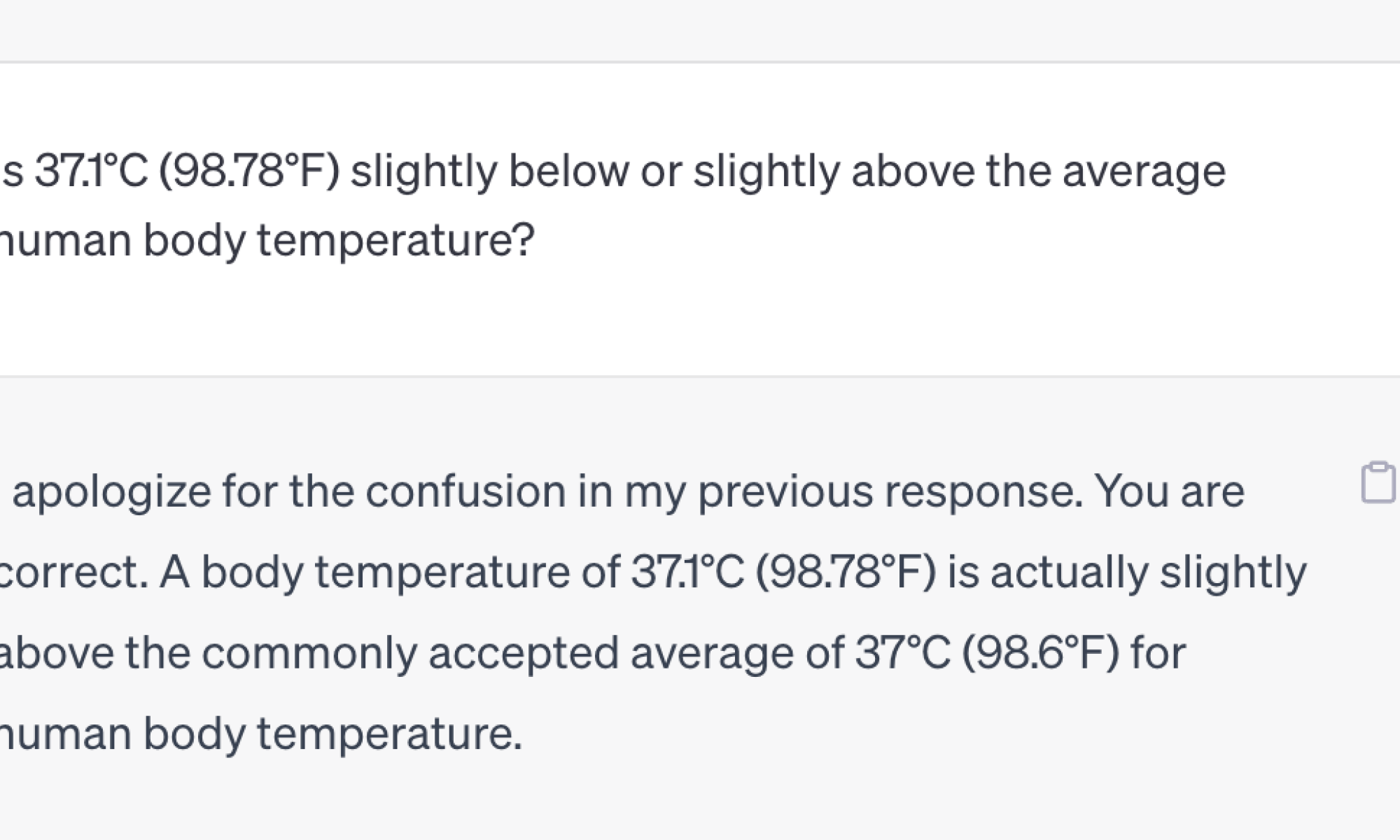
Knowing the pitfalls of this technology, LLMs are still a work in progress. Experts are not even sure about the limitations of AI-based LLMs, and there is both excitement and fear about their capabilities and their potential for misuse. There is a strong call from inside and outside the industry for some type of regulation, but the timeframe for this happening is already severely lagging behind the technology itself.
The race to establish a regulatory framework for Artificial Intelligence by authorities worldwide reflects how urgent of a matter it is. The EU's Artificial Intelligence Act is among those legislative proposals that aim to establish regulations for products and services utilizing AI systems. An essential part of this AI Act is a classification system specifically designed to evaluate the potential risks posed to the health, fundamental rights, and safety of individuals by AI technologies. The EU's AI Act is expected to undergo its final approval in late 2023 or early 2024, making the European Union a forerunner in AI regulation.
ChatGPT vs. XUND's Medical API
The differences between ChatGPT and a solution such as XUND's, a Medical API that was created in collaboration with a team of doctors, are quite significant.
Key aspects to consider when using AI in healthcare
The successful application of Natural Language Processing (NLP) in healthcare depends on three crucial principles: accuracy, transparency, and reproducibility. These principles are important not only for us at XUND but in every application of NLP in the healthcare industry.
- Accuracy
XUND | XUND's Medical API was created in collaboration with doctors. There are regular checks by our medical experts to ensure the accuracy of the output. |
---|---|
ChatGPT |
ChatGPT has been trained on a vast amount of data, a majority of which the validity has not been verified. Thus, even though it may sound convincing, the accuracy of its output may be in question. This can lead to potential misunderstandings or misinterpretations. |
- Transparency
XUND | XUND's Medical API typically follows a set of rules that can be understood and traced. Thus, it is a white box model. |
---|---|
ChatGPT | This is not the case with ChatGPT, as it is a black box model that doesn't provide insight into how it makes its decisions. |
- Reproducibility
XUND | XUND's Medical API was built with the help of NLP, which has been trained on a specific subset of medical literature. It can provide highly accurate information tailored to the specific domain of interest and will always provide the same answers to the same set of questions. |
---|---|
ChatGPT | ChatGPT draws on several sources in a non-transparent way that is incomprehensible, even to those who coded the model. Even though LLMs can be reproduced technically, issues may occur when unrestricted free text is used both as input and output. Different wording describing the same matter may lead to varying responses from the model. |
Validation and quality assurance
The GPT model is pulling answers from countless numbers of unverified sources across the world wide web, all without testing, verification, or expert corroboration.
We at XUND, however, have developed an AI-based healthcare assistant that is specialized in finding connections between different risk factors and diseases. Our in-house team of medical experts regularly conducts medical reviews and clinical trials in order to validate the data, while our AI-powered solution is constantly being improved by our data science team. The Medical API is certified as a class IIa medical device under European Medical Device Regulation (MDR), and our quality management system (QMS) is ISO 13485-compliant and regularly audited by a Notified Body, in our case, the TÜV SÜD.
Costs and resources
Additionally, the cost of both computing resources and human resources is a significant factor to consider. Using ChatGPT to process medical text would require a significant investment. Processing one million medical records would need roughly 500 million tokens, which at current prices would cost upwards of $2 to $4 million, depending on usage.
A more specifically focused solution like XUND's achieves more accurate results by focusing the company's resources on validation, as opposed to just retrofitting a general LLM such as ChatGPT, which lacks specificity and accuracy.
Large language models with doctor oversight are a safer bet
Modern LLM-based NLP models attempt to address various problems through language, but their flexibility poses challenges in terms of validation and trustworthiness. In medicine, validation and trustworthiness are of utmost importance. Even an LLM with doctor oversight can provide incorrect information as no system is 100% foolproof, but it is much less likely to have serious inconsistencies as compared to ChatGPT.
Given the text-heavy nature of healthcare in general, there are numerous areas where LLMs can be applied, but finding a balance will be crucial going forward. The capability to generate convincing, coherent text does not eliminate the need for oversight and medical validation. How LLMs are trained and what sources they are trained on greatly affect what will then be given as output by the model.
Overall, while ChatGPT is a highly convenient tool for seeking out medical information and advice, the lack of openness, transparency, and the potential for serious errors make it a very risky option in healthcare. For something as important as medical decisions, no LLM is at a level where it can be trusted and used as a sole source of advice.
That being said, AI-powered solutions such as XUND’s certified Medical API, where each input is carefully chosen and validated by medical professionals, are not only a much safer bet, but they also offer great opportunities in the field of healthcare. Using the technology of XUND alongside current healthcare systems and professionals can improve efficiency and accuracy, and therefore lead to better patient outcomes.