Natural language processing in healthcare & how XUND is contributing
At XUND, we’ve been extracting medical information from natural language text to improve our Medical API from the very beginning. In this insight, we’ll walk you through what natural language processing (NLP) is, how large language models (LLM), deep neural networks (DNNs), and machine learning completely disrupt healthcare, how patients and doctors interact with them, and the challenges of developing NLP models.
What is natural language processing?
NLP uses AI and deep learning methods to analyze unstructured data that comes from the natural language of humans. Currently, the most prominent example of a natural language processing application is the language model ChatGPT, which is capable of understanding, interpreting, and generating human language. At XUND, our NLP model analyzes the results of millions of medical publications using DNNs. Then, the model reads and structures the text so other models or computer programs can work with it.
For example, the information can be translated into actionable healthcare insights based on real-world evidence. This can, in turn, be used by healthcare providers to adjust patient treatments, identify any gaps in actions taken so far, or find ways of reducing risk.
NLP — the answer to structuring unstructured data
“The quality of data depends primarily on two things: whether it’s complete and whether it’s structured. One problem with the data quality in the context of traditional healthcare is that the type of documentation allows physician bias (e.g., in the facts they see worthy of documentation or not, expressions the physician uses differently than other physicians, etc.).” — Adrian Schiegl, Head of Data Science at XUND.
According to industry reports, about 80% of medical data is unstructured. Despite advances in machine learning, unstructured data is not usable by the vast majority of computer systems, making data management challenging for healthcare companies and resulting in much of the data in healthcare environments being ignored, lost, or forgotten.
NLP is the response to these challenges and can address ambiguity with findings rooted in real-world evidence. By using large language models, it sets out to quickly analyze the natural language used by healthcare professionals and thus assist them in their jobs.
What problems NLP can solve in healthcare
The dominant applications of NLP involve creating, understanding, and classifying clinical documentation and published research.
In healthcare today, a lot of the vital work to analyze and assess medical studies is done via manual reading carried out by the physicians or doctors themselves. As you can imagine, in healthcare, decisions often need to be made incredibly fast to ensure the patient is taken care of — analyzing many medical studies can take days, weeks, or even years for a human. In comparison, NLP tools can do so in minutes and assist healthcare professionals in making calculated, evidence-based decisions. These models are built on machine learning algorithms and neural networks that can analyze vast data sets and learn and improve as they are fed with data.
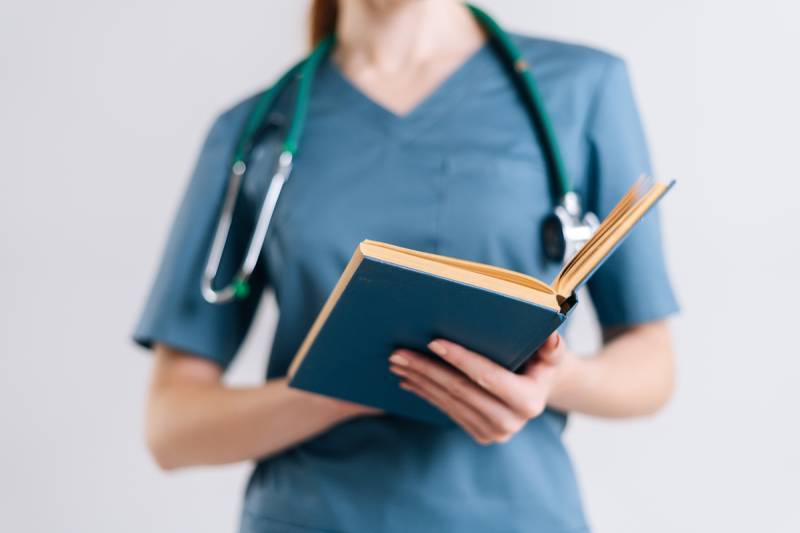
To us at XUND, it’s clear that natural language processing is the future of healthcare because it can:
- enhance patient treatment
- identify gaps in healthcare
- improve risk adjustment
- gather data for future drug research and personalized medicine
What are the challenges with developing an NLP product?
From our experience at XUND, developing NLP products comes with many challenges. The main one being that you need vast amounts of data to create a robust machine learning model. So the first challenge is gathering these enormous amounts of data. The second-biggest challenge is structuring and processing it to provide accurate and reliable information based on real-world evidence that healthcare professionals can use to improve their treatment plans.
“Data is something people confuse a lot with information, and they use both interchangeably even though they're very different. Data is actually just a signal — it becomes information when you interpret it and give it meaning. No one is really looking for data; they just want information, and that’s the essence of the data science team’s job here at XUND — to turn data into knowledge so people can take action based on the information we provide.” — Adrian Schiegl, Head of Data Science at XUND.
Want to find out more about the brains behind our natural language processing transformer models? Meet Adrian Schiegl, Head of Data Science at XUND, here.
Looking ahead: NLP & the future of XUND
Currently, XUND is leveraging NLP technology to analyze medical databases and unlock the knowledge from millions of medical publications for precise medical decision-making. Yet we continue striving to improve, gather even more data, and enhance our services.
XUND’s NLP research is funded by Austria Wirtschaftsservice (aws) under its Artificial Intelligence program and the Forschungsförderungsgesellschaft (FFG) under its General Program. Both project goals include using real-world data (such as discharge summaries) to extract patients’ clinical histories. We are proud of our role in contributing to scientific and medical progress by developing trustworthy, innovative AI projects in and for Austria.
With the FFG funding, we’re currently working on a joint research project (together with the Medical University of Graz, the Vienna University of Technology, and KAGes) to develop machine learning prediction models to improve outpatient care in hospitals.
We’ve also used aws’ support to expand the capabilities of our own NLP model and validate it against the English-language MIMIC database of the Massachusetts Institute of Technology (MIT). This vast database aggregates many aspects of patient information. We plan to integrate our learnings from this project with XUND’s core product, the Medical API, which has since been certified as a class IIa medical device.